The primary purpose of the ClimOP is to develop mitigation strategies for sustainable aviation. Several operational improvements are proposed to reduce the climate impact of aviation. Some of these improvements also have a direct impact on the flight schedules. When assessing the climate impact of these improvements, it is also essential to consider the impact of the schedule changes on the air transport network. Because of the snowball effect on the network, the schedule changes can lead to higher delays than expected. To analyse the impact of the schedule changes as a result of particular operation improvements, an airport-centric queuing network model is utilized.
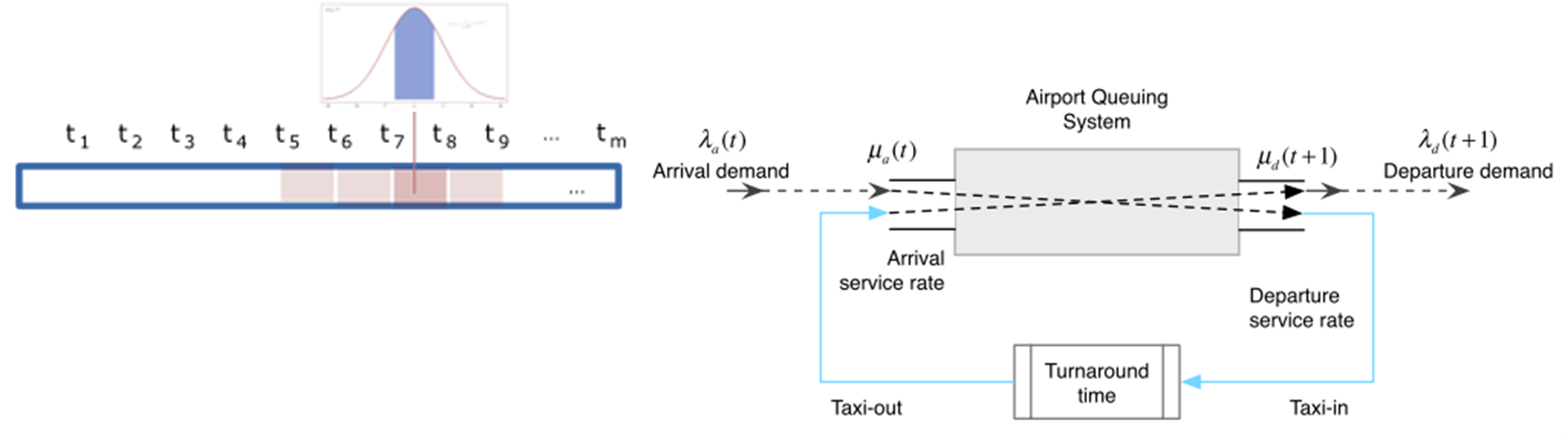
Figure 1. Airport Queues
Based on Queuing Theory, a data-driven model is proposed to propagate the given traffic through the network. The developed network model is used to assess delay/uncertainty propagation over the air transportation network with nominal or unconventional airport configurations (e.g., with reduced capacities, min or max turnaround times, etc.).
The model not only considers deterministic arrival traffic but also accepts the traffic with their probabilistic definitions. In this scope, airports are assumed as the elements of the network with their arrival and departure queues involving time delay for ground service. Figure 1 depicts the airport model with their arrival/departure queues and turnaround time.
The figure also demonstrates how the individual trajectories with their probability to be in the airport (or in the arrival slot) are used. In brief, the Queueing Network Model (QNM) aims to mimic the (time-dependent) dynamic behaviour of the traffic through the network for a given schedule and network-scale parametric uncertainties.
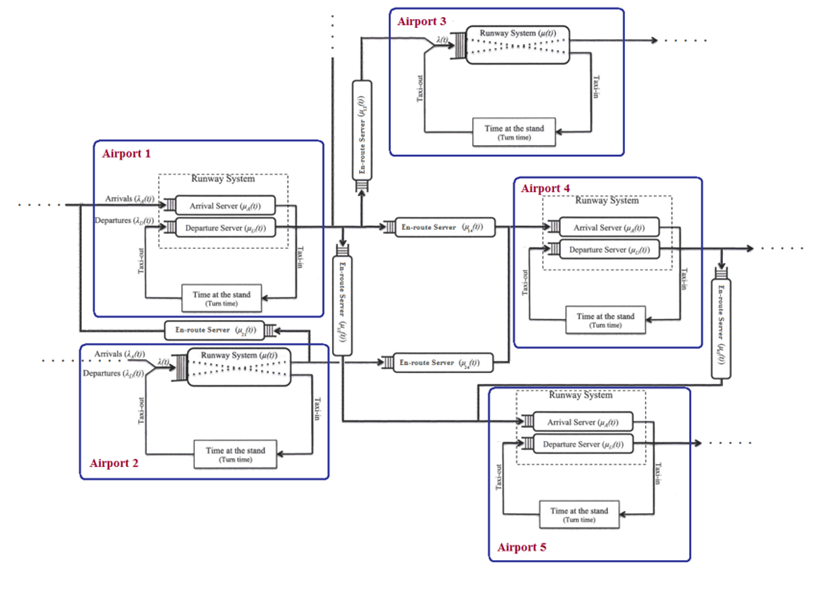
Figure 2. Airport-Centric Queueing Network Model
The queueing models applied to greener aviation
In queueing models, customers wait in a queue to get service according to their arrival times. Customers terminate their relationship with the system when the service is completed. Typically, a customer arrives, lines up for the queue, when the customer’s turn to come, receives the service, and finally leaves the system. However, when the system is a queueing network, the customer may move to another queue if planned. As it can be understood, queueing network models consist of various queues that are all connected. In queueing models, the considered important parameters are waiting/delay times in the system to determine actual demands and how they are distributed over a while.
The proposed network model, depicted in Figure 2, combines the airports to simulate the propagation of the given traffic over the network. The model allows injecting airport-related uncertainties based on their service rates and capacities. In the queueing network model, historical flight data is used to obtain the required parameters. The main parameters of a queue are inter-arrival times and service times, which correspond to the demand and capacity of the airport with their uncertainties. Inter-arrival times are defined according to flight schedules with their uncertainties. However, service time uncertainty is mainly related to the server, which is an airport in QNM.
In the model, the service time distribution of an airport is extracted using historical flights in saturation areas, which correspond to rush hours in the airport. For each airport, a gamma distribution is fitted to present the service time distribution. The gamma distribution parameters are calculated using the obtained service times (or samples) from the historical flight records with the help of the maximum likelihood estimation (MLE) method.
Author: Baris Baspinar, Instanbul Technical University